Found 21 talks width keyword Deep Learning
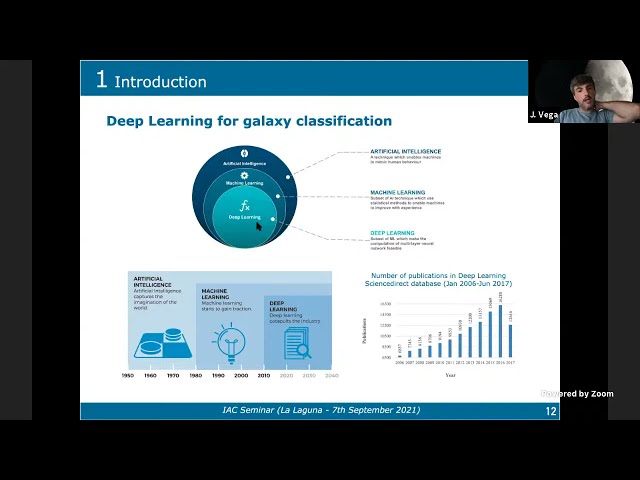
Abstract
Galaxy morphologies are one of the key diagnostics of galaxy evolutionary tracks, but visual classifications are extremely time-consuming. The sheer size of Big Data surveys, containing millions of galaxies, make this approach completely impractical. Deep Learning (DL) algorithms, where no image pre-processing is required, have already come to the rescue for image analysis of large data surveys. In this seminar, I will present the largest multi-band catalog of automated galaxy morphologies to date containing morphological classifications of ∼27 million galaxies from the Dark Energy Survey. The classification separates: (a) early-type galaxies (ETGs) from late-types (LTGs); and (b) face-on galaxies from edge-on. These classifications have been obtained using a supervised DL algorithm. Our Convolutional Neural Networks (CNNs) are trained on a small subset of DES objects with previously known classifications, but hese typically have mr < 17.7 mag. We overcome the lack ofa training sample by modeling fainter objects up to mr < 21.5 mag, i.e., by simulating what thebrighter objects with well-determined classifications would look like if they were at higher redshifts.The CNNs reach a 97% accuracy to mr < 21.5 on their training sets, suggesting that they are ableto recover features more accurately than the human eye. We obtain secure classifications for 87%and 73% of the catalog for the ETG vs. LTG and edge-on vs. face-on models, respectively.
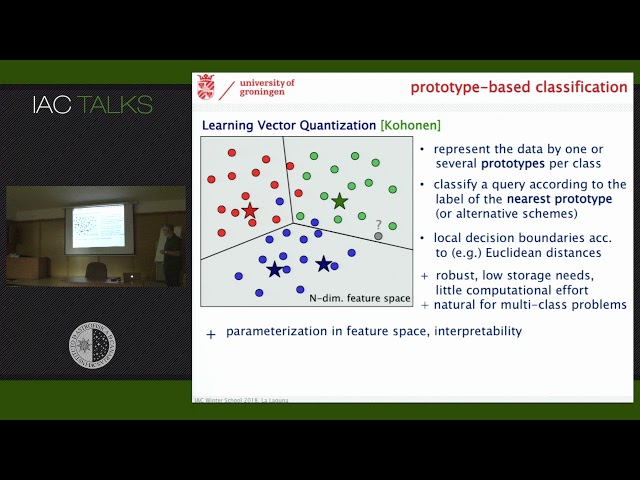
Abstract
Series: XXX Canary Islands Winter School of Astrophysics: Big Data in Astronomy
Topic: Supervised learning: classification and regression
Lecture 4
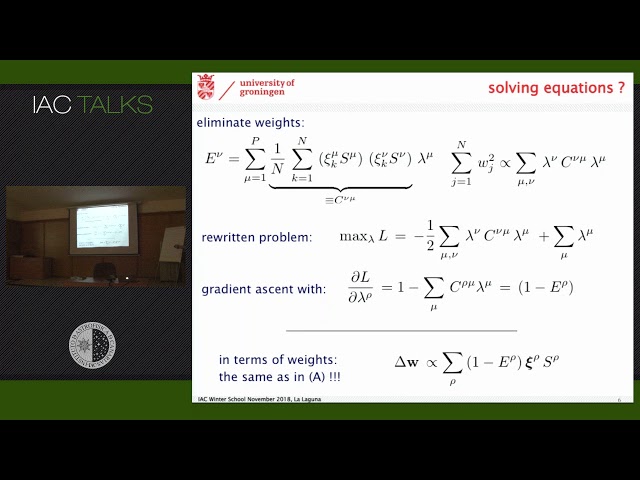
Abstract
Series: XXX Canary Islands Winter School of Astrophysics: Big Data in Astronomy
Topic: Supervised learning: classification and regression
Lecture 3
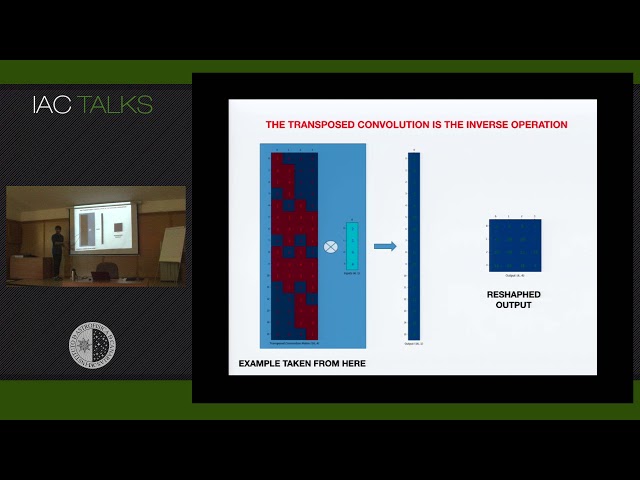
Abstract
Series: XXX Canary Islands Winter School of Astrophysics: Big Data in Astronomy
Topic: Deep learning
Lecture 4
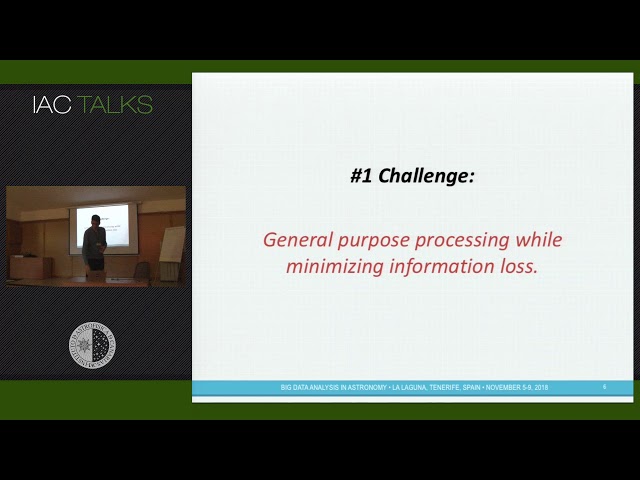
Abstract
Series: XXX Canary Islands Winter School of Astrophysics: Big Data in Astronomy
Topic: Data challenges and solutions in forthcoming surveys
Lecture 4
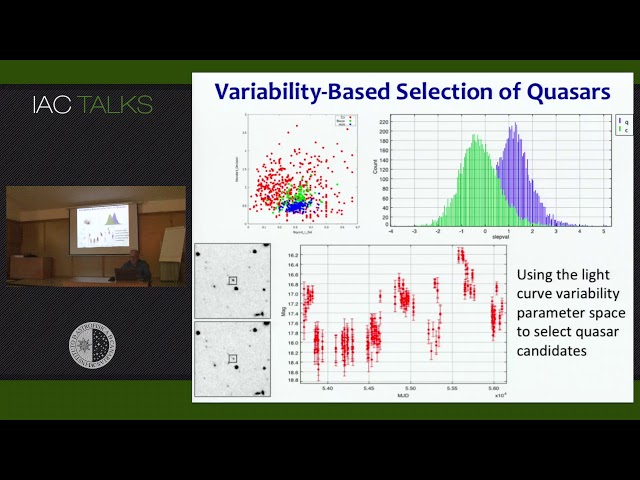
Abstract
Series: XXX Canary Islands Winter School of Astrophysics: Big Data in Astronomy
Topic: General overview on the use of machine learning techniques in astronomy
Lecture 4
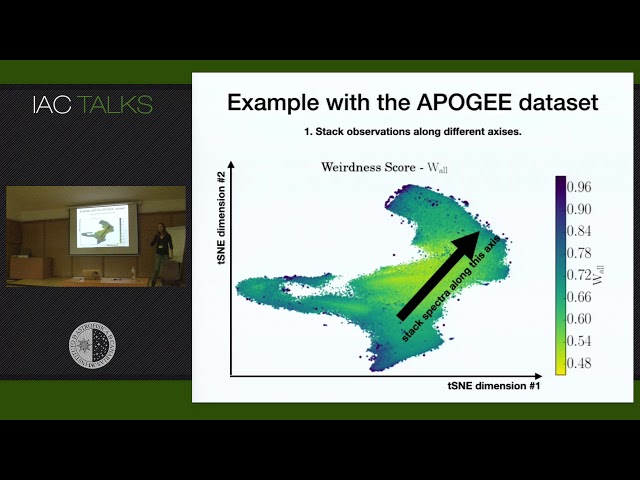
Abstract
Series: XXX Canary Islands Winter School of Astrophysics: Big Data in Astronomy
Topic: Machine learning methods for non-supervised classification and dimension reduction techniques
Lecture 4
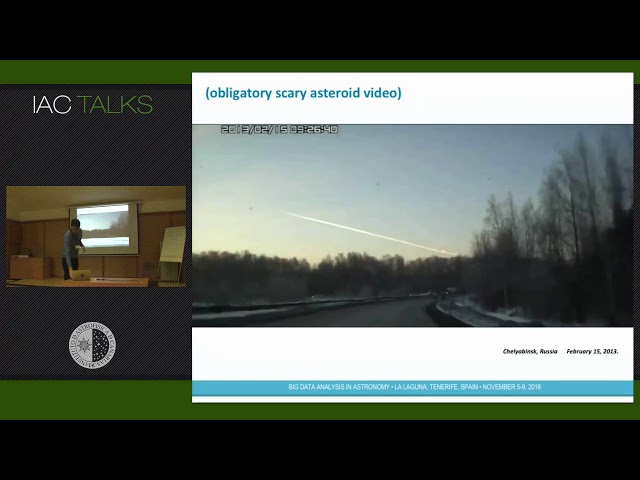
Abstract
Series: XXX Canary Islands Winter School of Astrophysics: Big Data in Astronomy
Topic: Data challenges and solutions in forthcoming surveys
Lecture 3
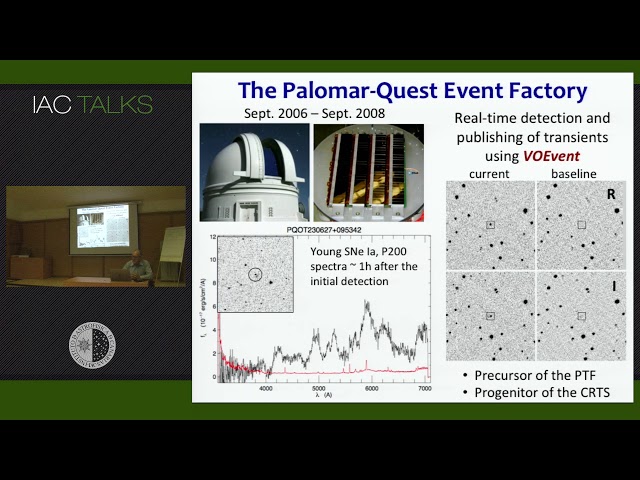
Abstract
Series: XXX Canary Islands Winter School of Astrophysics: Big Data in Astronomy
Topic: General overview on the use of machine learning techniques in astronomy
Lecture 3
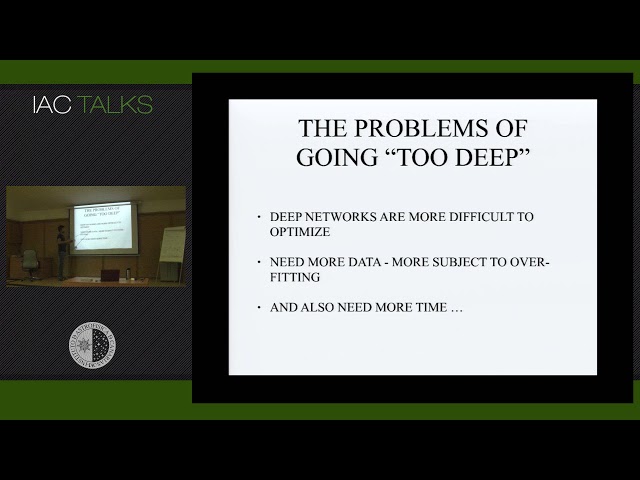
Abstract
Series: XXX Canary Islands Winter School of Astrophysics: Big Data in Astronomy
Topic: Deep learning
Lecture 3
No sound available since minute ~5.
« Newer 1 | 2 | 3 Older » Last >>
Upcoming talks
No talks scheduled for the next days.